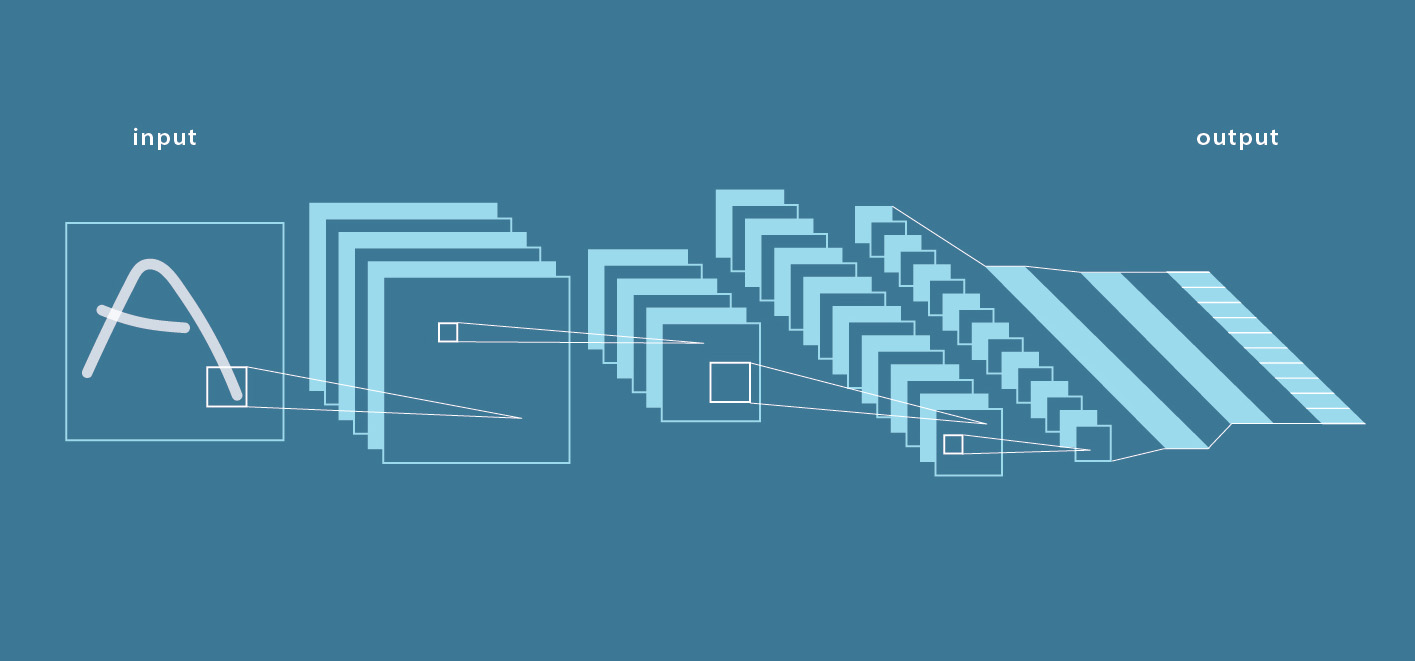
Machine Learning
Faculty
- Courant Institute: Joan Bruna, Kyunghyun Cho, Eunsol Choi, Sumit Chopra, Ernest Davis, Rob Fergus, Carlos Fernandez-Granda, David Fouhey, Yanjun Han, He He, Arthur Jacot, Yann LeCun, Qi Lei, Mehryar Mohri, Daniel Neill, Jonathan Niles-Weed, Benjamin Peherstorfer, Lerrel Pinto, Jean Ponce, Rajesh Ranganath, Oded Regev, Mengye Ren, MatusTelgarsky, Eric Vanden-Eijnden, Andrew Wilson, Saining Xie;
- Faculty of Arts and Science: Samuel Bowman, Brenden Lake, Weiji Ma;
- NYU Grossman School of Medicine: Sumit Chopra, Krzysztof Geras;
- Robert F. Wagner Graduate School of Public Service: Daniel Neill;
- NYU Abu Dhabi: Keith Ross, Julian Togelius;
- Stern School of Business: Xi Chen;
- Tandon School of Engineering: Anna Choromanska, David Fouhey, Guido Gerig, Chinmay Hegde, PavelIzmailov (Starting Fall 2024), Christopher Musco, Keith Ross, Julian Togelius, Eugene Vinitsky;
- Center for Data Science: Samuel Bowman, Joan Bruna, Kyunghyun Cho, Eunsol Choi, Carlos Fernandez-Granda, Yanjun Han, He He, Brenden Lake, Qi Lei, Jonathan Niles-Weed, Jean Ponce, Rajesh Ranganath, Mengye Ren, Andrew Wilson;
-
Algorithms and Foundations Group
Our group is composed of researchers interested in applying mathematical and theoretical tools to a variety of disciplines in computer science, from machine learning, to systems, to geometry, to computational biology, and beyond.
-
Computational Intelligence, Learning, Vision, and Robotics (CILVR)
The CILVR Lab (Computational Intelligence, Learning, Vision, and Robotics) regroups faculty members, research scientists, postdocs, and students working on AI, machine learning, and a wide variety of applications, notably computer perception, natural language understanding, robotics, and healthcare.
-
Control and Network Lab
Research activities in the Control and Network (CAN) Lab mainly focuses on the development of fundamental principles and tools for the stability analysis and control of nonlinear dynamical networks, with applications to information, mechanical and biological systems.
-
Data for Intelligent Computing and Engineering Lab
The Data for Intellingent Computing and Engineering Lab currently focuses on the following aspects of Machine Learning:Theoretical and applied aspects of Inverse Problems using Deep Learning, Physics-informed Machine Learning, Adversarial Machine Learning, Deep Reinforcement Learning, Distributed Machine Learning, and Autonomous Navigatio
-
Human and Machine Learning Lab
Today’s AI provides nothing like the general purpose, flexible intelligence that we have as humans. We are studying the ingredients of intelligence that enable fast and flexible learning
-
Machine Learning for Good
Our lab is focused on development of novel machine learning methods for addressing critical urban problems. By creating, deploying, and evaluating new methods in collaboration with public sector partners, we hope both to advance the state of the art in machine learning and to improve the quality of public health, safety, and security.
-
Machine Learning for Language
The Machine Learning for Language (ML²) group is a team of researchers at New York University working on developing and applying state-of-the-art machine learning methods for natural language processing (NLP), with a special focus on artificial neural network models. ML² is affiliated with the larger CILVR lab.
-
Math and Data
The Mad (Math and Data) group studies the fundamental mathematical structures underpinning modern data analysis, machine learning, deep learning, and their applications.
-
STAT Research Group
The STAT group (Statistics: Tools, Algorithms, and Theory) seeks to advance the state-of-the-art in statistics, by developing new methodological, computational, and mathematical approaches to statistical problems and to their applications in data science and machine learning. The STAT group assembles faculty, fellows and students across CDS and the Courant Institute.