About the Authors
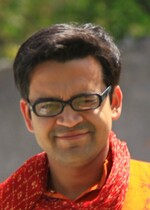
Deeparnab Chakrabarty
Researcher
Microsoft Research
9 Lavelle Road, Bangalore, India, 560001
deeparnab[ta]gmail[td]com
research.microsoft.com/en-us/um/people/dechakr/
Researcher
Microsoft Research
9 Lavelle Road, Bangalore, India, 560001
deeparnab[ta]gmail[td]com
research.microsoft.com/en-us/um/people/dechakr/
Deeparnab Chakrabarty received his
B. Tech. from IIT Bombay in 2003 and Ph.D. from Georgia Institute of Technology
in 2008. His graduate adviser was
Vijay V. Vazirani.
After stints at the universities of
Waterloo
and Pennsylvania, he
crossed the pond again to
join Microsoft Research in Bangalore where he has been a researcher since 2011.
He is interested in understanding efficient algorithms using the lens of
optimization and has worked on approximation algorithms, property testing,
and on algorithmic questions arising in game theory and economics.
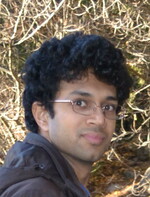
C. Seshadhri
Assistant Professor
Department of Computer Science
University of California, Santa Cruz
scomandu[ta]ucsc[td]edu
csesha[ta]gmail[td]com
https://users.soe.ucsc.edu/~sesh/
Assistant Professor
Department of Computer Science
University of California, Santa Cruz
scomandu[ta]ucsc[td]edu
csesha[ta]gmail[td]com
https://users.soe.ucsc.edu/~sesh/
C. Seshadhri (Seshadhri Comandur according to his passport, and Sesh
according to his friends) follows the common naming style of South India,
the native land of his parents. In three of his early papers, including
one cited in this bibliography, his name appears as S. Comandur. The
interested reader is invited to consult the
OAQ (Occasionally Asked
Questions) section of his website for more details. Sesh
got his B. Tech. from IIT Kanpur in 2003 and
his Ph.D. from Princeton University
in 2008.
His Ph.D. advisor was Bernard Chazelle.
He spent two years at IBM Almaden,
and in 2010 he became a member of technical staff at Sandia National
Laboratories, Livermore, California.
In 2015 he joins
the faculty of the University of
California at Santa Cruz. This paper was written during his time at Sandia.
His research focuses on how random sampling can be used for algorithms
for massive inputs.
In theory, this manifests
itself as work in property testing. In practice, this
has led to research on algorithms
for massive real-world graphs. He is increasingly interested in algorithmics
beyond the worst-case,
and bridging the gap between theory and practice for big data applications.