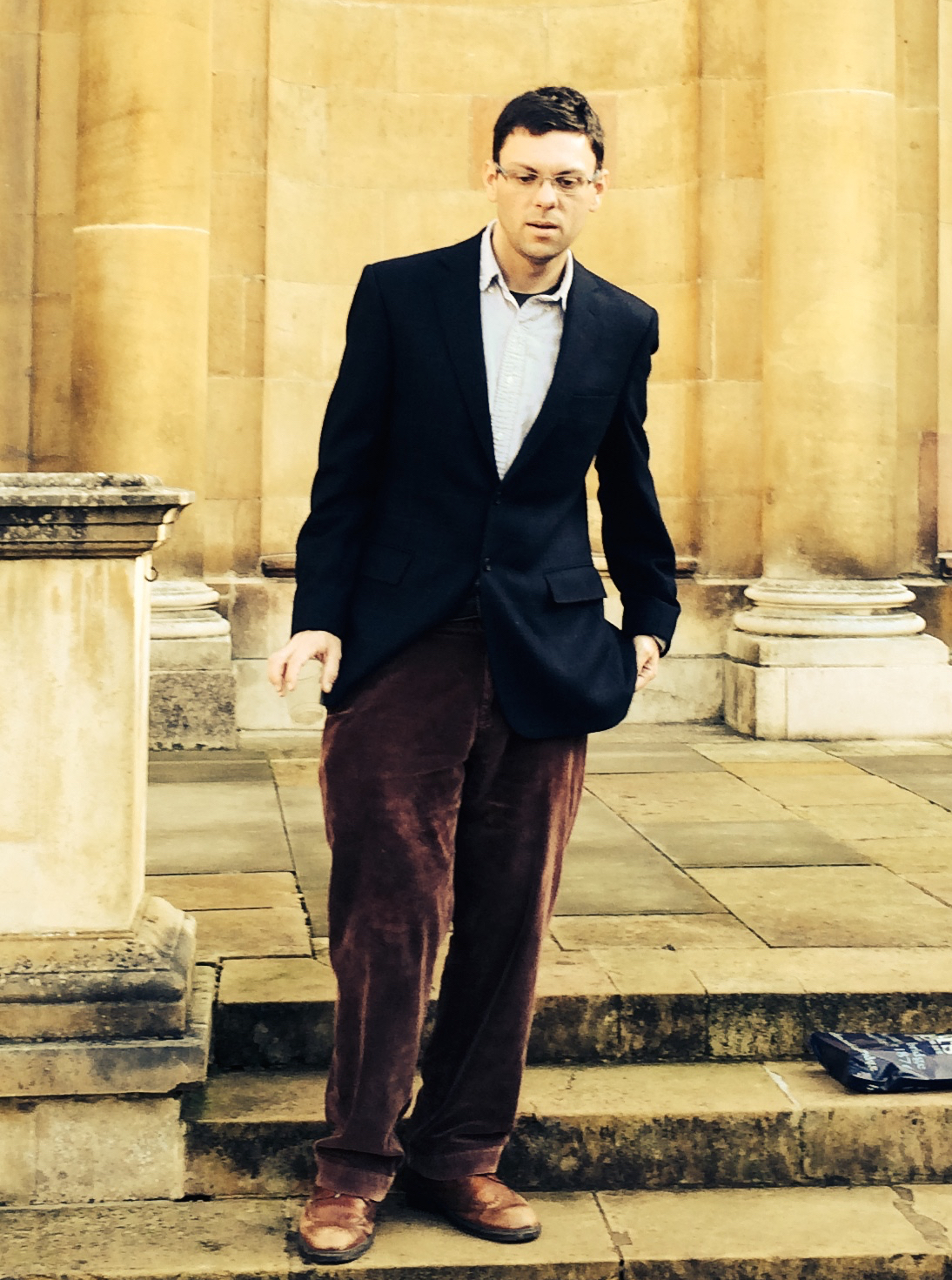
Andrew
Gordon Wilson
We are a research group at New York
University, wishing to understand the foundations of
generalization, learning, and decision making, towards building highly practical new methods in machine learning. Our work covers deep learning models, including LLMs, uncertainty representation, AI alignment, distribution shifts, physics inspired ML, equivariance modelling, numerical methods, and scientific discovery.
We believe in open and reproducible research. If
you'd like to try out these methods check out our code
page. See also our info about joining, which describes research interests and some example papers in greater detail.
Group Leader
Andrew Gordon Wilson
Postdoctoral Fellows
Alan Amin
Zhe Zeng
PhD Students
Ethan Baron
Zixi (Charlie) Chen
Marc Finzi
Nate Gruver
Sanyam Kapoor
Yucen (Lily) Li
Martin Marek
Sanae Lotfi
Andres Potapczynski
Hoang Phan
Shikai Qiu
Alumni
Ben Athiwaratkun (Scientist at Together AI)
Greg Benton (Scientist at Celonis)
Ian Delbridge (Senior Research Scientist at Klaviyo)
Marc Finzi (Postdoc at CMU)
Jacob Gardner (Assistant Professor at the University of Pennsylvania)
Micah Goldblum (Assistant Professor at Columbia University)
Pavel Izmailov (Scientist at Anthropic, and incoming Assistant Professor at NYU)
Polina Kirichenko (Research Scientist at FAIR Labs, Meta)
Wesley Maddox (Quantitative Researcher at Jump Trading)
Geoff Pleiss (Assistant Professor at the University of British Columbia)
Samuel Stanton (Research Scientist at GenenTech)
Ke Alexander Wang (PhD Student at Stanford)
Ruqi Zhang (Assistant Professor at Purdue University)